event-triggered系列论文学习
Paper 1:Event-Triggered Real-Time Scheduling of Stabilizing Control Tasks, 2007, TAC
一、motivation
传统的固定时间周期的采样方式缺乏严格量化证明(时间周期为多少合适?少了不准确,多了浪费资源),而在微型处理器上又希望能尽可能节约计算资源, 所以考虑将周期采样转换为非周期采样,采样时间由某个“事件”来触发。
二、预备知识
- $k$类函数、$k_\infty$类函数
如果连续函数$\alpha:[0,a) \rightarrow [0,\infty)$严格递增,且$\alpha(0)=0$,则$\alpha$属于$k$类函数;更进一步地,如果$a=\infty$,当$r\rightarrow \infty$时,$\alpha®\rightarrow\infty$,那么$\alpha$属于$k_\infty$类函数
-
问题描述
控制系统:$\dot x=f(x,u)$
反馈控制:$u=k(x)$
恒定控制:$t\in[t_i+\Delta,t_{i+1}+\Delta]\Longrightarrow u(t)=u(t_i+\Delta)$(Δ是现实中的额外耗时,IO读取、计算耗时之类的)
测量误差:$t\in[t_i+\Delta,t_{i+1}+\Delta]\Longrightarrow e(t)=x(t_i)-x(t)$
-
ISS Lyapunov函数
其中$\underline\alpha,\alpha,\overline\alpha,\gamma$都是$k_\infty$类函数
三、event-triggered 机制
-
如果我们设置有关误差的约束条件为:
$$
\gamma(|e|)\leq\sigma\alpha(|x|),\quad\sigma>0\quad\quad(8)
$$
将其代入公式 (5) 可得:
$$
\frac{\partial V}{\partial x}f(x,k(x+e))\leq(\sigma-1)\alpha(|x|)
$$
那么此时我们只需要让$\sigma<1$就可保证Lyapunov函数 V 是递减的。因此,我们可以将事件触发规则设置为下式:
$$
\gamma(|e|)=\sigma\alpha(|x|)\quad\quad(9)
$$ -
在设置好触发规则之后,一个相应的问题是:触发时间由 (9) 隐式定义,那如何保证两次触发时间不会无限接近,从而造成芝诺行为呢?
原文通过Lipschitz条件证明了只要时延Δ足够小,则最小执行间隔时间存在,也就保证了$t_i-t_{i+1}$的下界,即$t_i-t_{i+1}\ge\tau,\tau\in\mathbb{R}^{+}$
具体证明过程略
-
仿真代码
$$
\left[\begin{matrix}\dot{x}_1\\
\dot{x}_2\end{matrix}\right]=\left[\begin{matrix}0&1\\
-2&3\end{matrix}\right]\left[\begin{matrix}x_1\\
x_2\end{matrix}\right]+\left[\begin{matrix}0\\
1\end{matrix}\right]u
$$$$
V=x^TPx
$$$$
\begin{aligned}\partial V/\partial x(Ax+BKx)=-x^{T}Qx\end{aligned}
$$$$
P=\left[\begin{array}{cc}1&\frac{1}{4}\\
\frac{1}{4}&1\end{array}\right],\quad Q=\left[\begin{array}{cc}\frac{1}{2}&\frac{1}{4}\\
\frac{1}{4}&\frac{3}{2}\end{array}\right]
$$把测量误差带入表达式,我们有:
$$
\frac{\partial V}{\partial x}\Big(Ax+BKx+BKe\Big)\leq-a|x|^2+b|e|x|
$$
其中
$$
a=\lambda_m(Q)>0.44\quad b=|K^TB^TP+PBK|=8
$$
$\lambda_m(Q)$表示 Q 的最小特征值,$\sigma b$应比0.44更小,算出来$\sigma$的上界约为0.05,我们可以取$\sigma’=0.03$1
2
3
4
5
6
7
8
9
10
11
12
13
14
15
16
17
18
19
20
21
22
23
24
25
26
27
28
29
30
31
32
33
34
35
36
37
38
39
40
41
42
43
44
45
46
47
48
49
50
51
52
53
54
55
56
57
58
59
60
61
62
63
64
65
66
67
68
69
70
71
72
73
74
75
76
77
78
79
80
81% Tabuada, P. (2007).
% Event-triggered real-time scheduling of stabilizing control tasks.
% IEEE Transactions on Automatic Control,52(9), 1680-1685.
clc;
clear;
close all;
%% system define
A = [0 1; -2 3];
B = [0; 1];
K = [1 -4];
P = [1 0.25; 0.25 1 ];
Q = [0.5 0.25; 0.25 1.5];
x0 = [0.5 0.5]';
dt = 0.001;
trigger_count = 0;
% sigma是上界,sigma_prime应小于sigma
% 原论文中有解释
sigma_prime = 0.03;
sigma = 0.05;
%% simulation
x_buffer = [];
u_buffer = [];
error_buffer = [];
error_trigger = [];
error_upper_bound = [];
x = x0;
u = K*x0;
syms s
% 定常连续系统方程的离散化
% 具体计算原理可参考https://zhuanlan.zhihu.com/p/556915351的2.2节
Ad = expm(A*dt);
Bd = int(expm(A*s),0,dt)*B;
Bd = eval(Bd);
for step = 1:15000
error = x - x0;
% 欧几里得范数也是一种k类函数
if norm(error) >= sigma_prime * norm(x)
x0 = x;
u = K*x0;
trigger_count = trigger_count + 1;
end
x = Ad*x + Bd*u;
E = norm(error);
ET = sigma_prime * norm(x);
EUB = sigma * norm(x);
error_buffer = [error_buffer E];
error_trigger = [error_trigger ET];
error_upper_bound = [error_upper_bound EUB];
x_buffer = [x_buffer x];
u_buffer = [u_buffer u];
end
%% plot
figure(1)
hold on
plot(x_buffer', 'LineWidth', 1.5);
plot(u_buffer, 'LineWidth', 1.5);
legend('x1', 'x2', 'u');
figure(2)
hold on
plot(error_buffer, 'LineWidth', 1);
plot(error_trigger, 'LineWidth', 1.5, 'Color', 'r');
plot(error_upper_bound, 'LineWidth', 1, 'Color', 'r');
legend('error', 'error trigger', 'error upper bound');
fprintf('the system has been triggered %d times in all\n', trigger_count);仿真结果:
可见最后的实际误差确实在由事件触发规则限制的误差之下,系统也达到了收敛状态
Paper 2:Distributed Event-Triggered Control for Multi-Agent Systems, 2012, TAC
一、motivation:
- 上一篇文献没提”多智能体“,讲的只是一个普通的控制系统的事件触发;而这篇文章讲的MAS中的事件触发
- 分为集中式(各个节点都知道全局信息,如拉普拉斯矩阵)和分布式(各节点只知道自己的邻居信息)
- 给出了自触发公式:算出两次触发时间之间的上限,只要间隔不超过这个上限最后就能稳定
二、集中式事件触发
模型:单积分器 $\dot{x_i}=u_i$
控制器:$u=-Lx$
拓扑关系:无向连通图
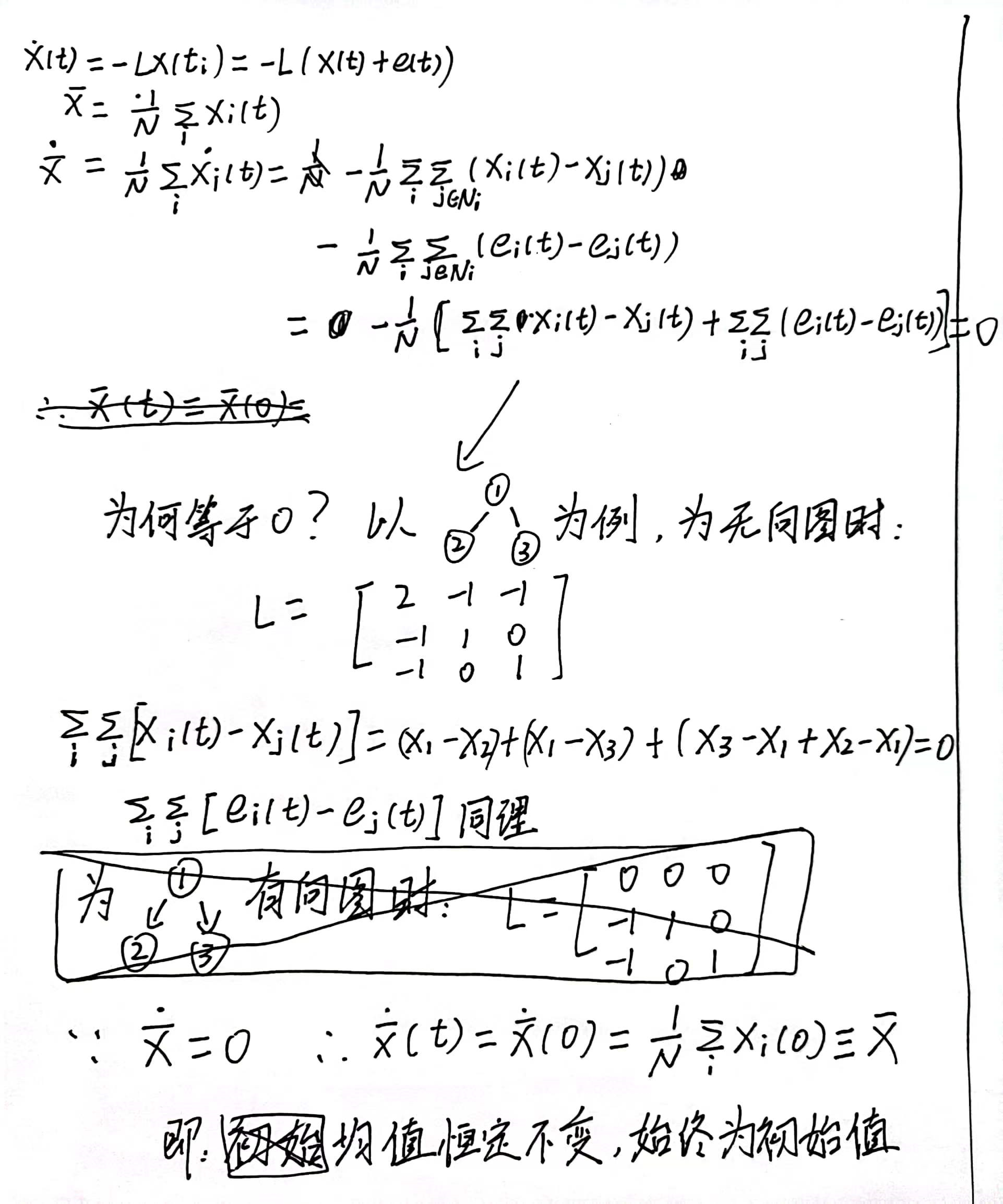
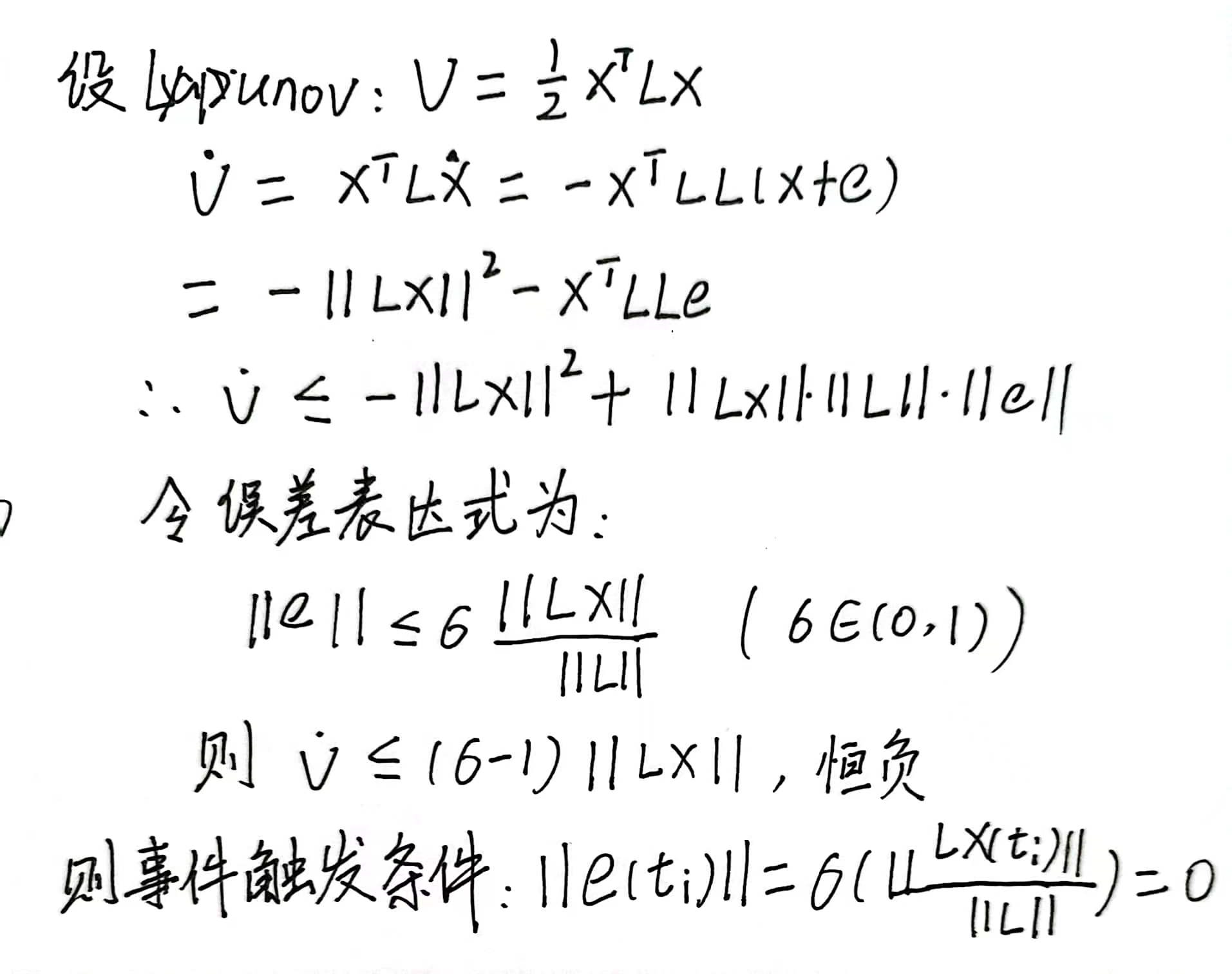
由上述推导可知,事件触发条件为:
$$
||e||=\sigma\frac{||Lx||}{||L||}
$$
二、分布式事件触发
基本思路是每个智能体都只能获取邻居信息,无法得知全局信息(Laplace矩阵);哐哐一通推导后得到每个智能体的事件触发条件:
$$
e_i^2=\frac{\sigma_ia(1-a|N_i|)}{N_i}z_i^2
$$
三、自触发
idea:推导出两次触发时间的间隔上界,即只要时间间隔别超过这个上界就能稳定;在自触发中,“事件”不再由误差e决定,而是取决于间隔时间
$$
\begin{aligned}\Delta&=4\sigma^4\left|(Lx(t_i))^TLLx(t_i)\right|^2\&+4\sigma^2\left|L^2x(t_i)\right|^2\cdot\left(|Lx(t_i)|^2|L|^2-\sigma^2\left|L^2x(t_i)\right|^2\right)>0.\end{aligned}
$$
$$
t_{i+1}-t_i\leq\frac{-2\sigma^2\left(Lx(t_i)\right)^TLLx(t_i)+\sqrt{\Delta}}{2\left(|Lx(t_i)|^2|L|^2-\sigma^2|L^2x(t_i)|^2\right)}
$$
四、仿真
1 |
|